Professor Christina Messiou talks us through her study that shows AI is nearly twice as good at grading the aggressiveness of a rare form of cancer from scans as the current method.

The onward march of medical technology recorded another key achievement in November with the publication in The Lancet Oncology of the results from a study that tested the ability of machine learning to predict the type and grade of soft tissue cancers in patients.
The article – “A CT-based radiomics classification model for the prediction of histological type and tumour grade in retroperitoneal sarcoma (RADSARC-R): a retrospective multicohort analysis” – describes the outcome of a joint research project by a team from The Royal Marsden NHS Foundation Trust and the Institute of Cancer Research and that worked on patient data from UK hospitals and several medical institutions across Europe and the US.
Seeds of the work
A lead author of the article, Professor Christina Messiou, Consultant Radiologist at The Royal Marsden and Team Leader at The Institute of Cancer Research, says the seeds of the work were planted during her time as Imaging Lead for the EORTC Soft Tissue and Bone Sarcoma Group. “I was involved in the STRASS trial, the primary aim of which was to assess whether radiotherapy would be beneficial for patients with retroperitoneal sarcomas.
I recognised that as a unique opportunity to bring together valuable data from many different centres on a rare cancer type and that we could use to achieve even more benefit for patients.”
“Radiomics can define features, which are difficult for the human eye to see”
In addition to the STRASS data, The Royal Marsden, as one of the key global centres for researching and treating sarcoma units, could call on its own extensive bank of patient data. With that spark of an idea, Messiou began to think how that data might be used to help patients. “One of the substantial areas of unmet need was grading retroperitoneal sarcomas accurately prior to surgery. Treatment decisions are based on the grade of the tumour, and if we get that wrong we can potentially under-treat. The other challenge is diagnosing these rare cancers accurately in the first place. So those were the two questions that we prioritised.”
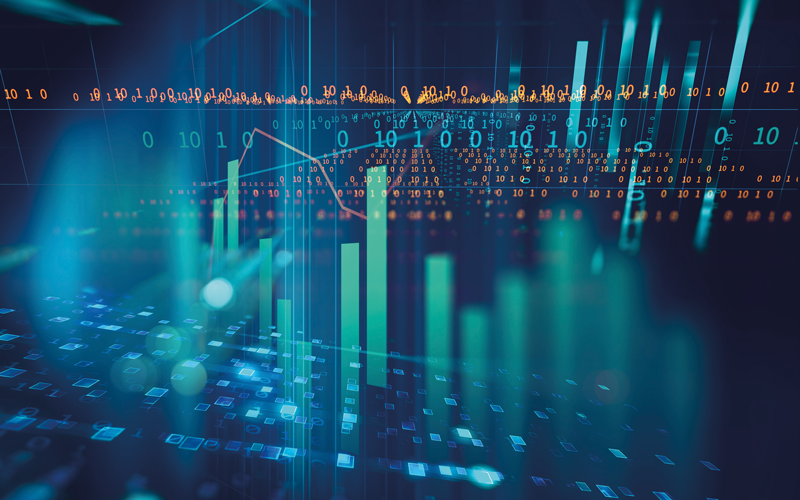
Difficult to diagnose
Sarcomas are prime subjects for investigation, says Messiou, because they present big challenges. “Firstly, they’re rare, so they can go undiagnosed for a long time. It can be difficult to identify sarcomas. The second challenge is that there are many different subtypes of sarcoma. They can occur almost anywhere in the body and so one treatment doesn’t fit all. They can be very difficult tumours to diagnose and treat.”
To meet these challenges, the team extracted data from CT scans using an AI technique known as radiomics. Could this technique identify and classify the more subtle characteristics of sarcoma?
“As a consultant radiologist, I normally look at a patient’s CT scan as a picture,” says Messiou. “So my reports for a scan essentially describe what I see. Radiomics allows us to turn those pictures into data, or numerical features. At the basic end of the spectrum are recognisable features that relate to the size and shape of the cancer, or the margins of the tumour. But at the other end of the spectrum, radiomics can define features such as the texture of the tumour, which are difficult for the human eye to see and certainly difficult for me to articulate verbally. This opens up a more consistent and scientific approach to analysing scans.”
Explainable AI
Radiomics is a technique that has developed rapidly over the past decade, but Messiou and the team took a novel approach by adding an intervention, based on their understanding of the biology of the cancers, to the algorithm. “We were aiming for more explainable AI. One of the reasons we are so optimistic about the outcomes of the study is that we have insights into how the algorithm is making its decisions, which is reassuring.” Another reassuring point is that the algorithm has been validated on CT scans from several different hospitals, all of which may produce their data in slightly varying ways – the algorithm has absorbed these potential variations and, as a result, is not limited to data from a single site.
But little of this would be hugely remarkable if the algorithm hadn’t proved its worth in completing its assignment. “In grading the aggressiveness of tumours, the algorithm was twice as good as the percutaneous core needle biopsy,” says Messiou. “In terms of accuracy, it was 82% with the radiomics compared to 44% with the biopsy. That was a tremendous uplift in accuracy.”
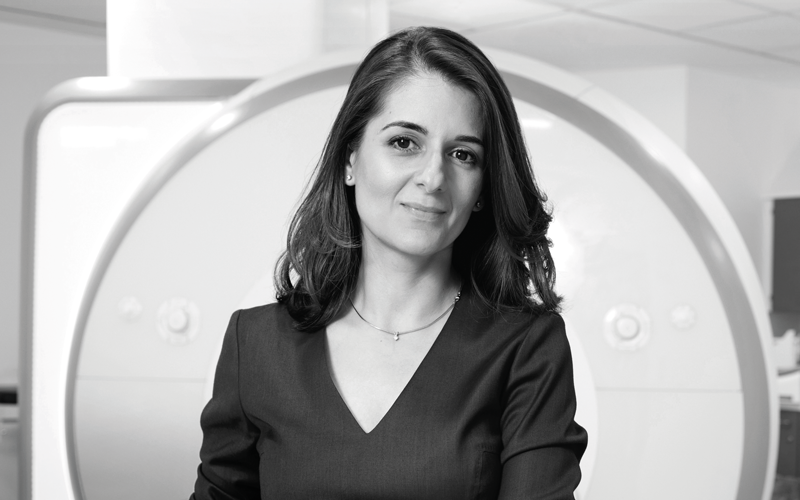
Prof. Messiou
- 1998: BMedSci; BMBS, the University of Nottingham
- 2011: MD(Res), the Institute of Cancer Research
- 2007: Imaging Fellow, the Institute of Cancer Research
- 2011: Consultant Radiologist, The Royal Marsden NHS Trust
- 2014: Roentgen Professor, The Royal College of Radiologists
- 2022: Professorship, the Institute of Cancer Research.
Machine-learning operations
The next step for the research is to assess how the algorithm performs over time. “Monitoring the performance of algorithms is a critical task, and we are just in the process of building what we call ‘machine-learning operations’, which will allow us to run the algorithm in parallel with our routine clinical workflow and to assess its performance over time. It’s not until we’ve done that we would start to think about using this to support our clinical decision-making for patients.”
The potential clinical benefits for patients lie in the quick and accurate grading of these often-elusive tumours, which will, in turn, better inform the selection of targeted treatment, leading to more positive outcomes. In future, says Messiou, it would be reasonable to expect that some patients could be offered a virtual biopsy. “The patients I think would be offered this first are those for whom the biopsy is too risky. Some may have lesions in locations where it’s just not safe to offer a biopsy, and therefore planning treatment is really challenging.”
Exciting possibilities
As the beginning of a new diagnosis and treatment paradigm for these rare cancers, the research offers exciting possibilities. What would Messiou hope it might achieve in 10 years’ time? “In terms of next-generation thinking, our ambition is to use AI to merge information from CT scans and tissue samples, and create the next generation of biomarkers. We want to make the data more valuable than the sum of its parts, and to bring together data from different modalities we will need AI.”
Image credit | iStock | Dominick-Tyler